B2B credit scoring: the limits of traditional banking models
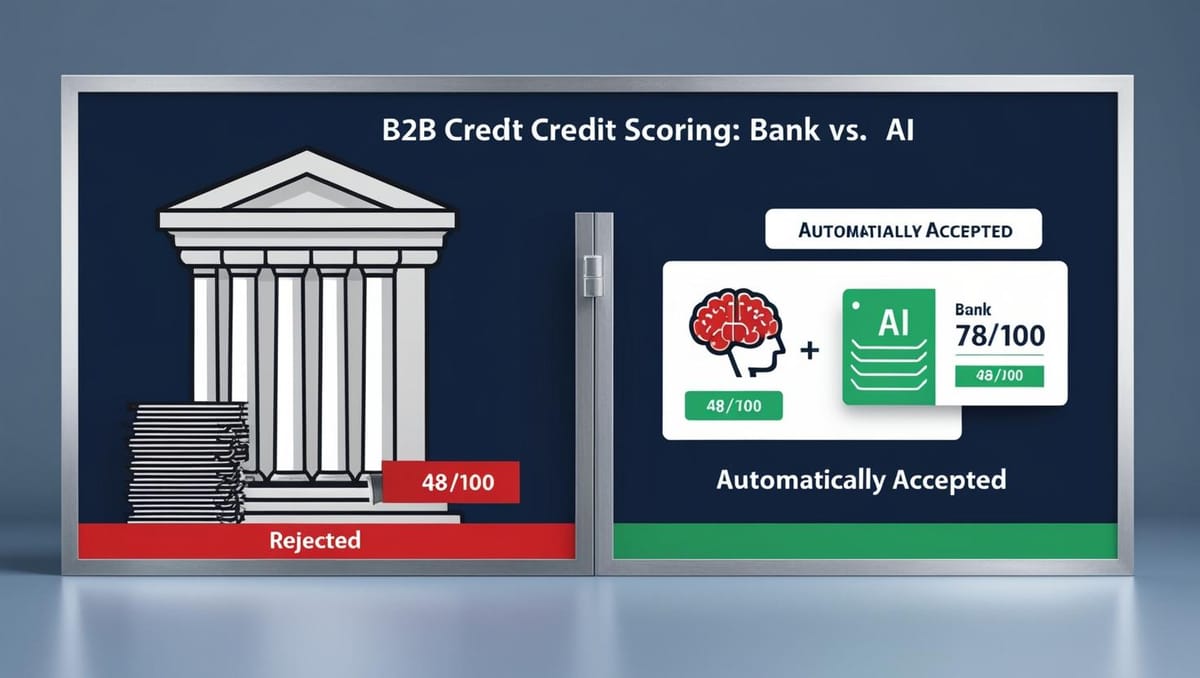
For decades, banks and credit insurers have been using standard scoring models to assess the creditworthiness of companies.
But are these models, conceived in the pre-digital era, still adapted to the diversity of B2B companies?
More and more often, the answer is no.
In this article, we explore :
- Why conventional models fail to properly assess VSEs/SMEs
- How modern AI-based approaches are transforming scoring
- And how RocketFin helps build a fairer, more dynamic and operational score.
Why traditional banking models penalize SMEs
🧾 Historical criteria... unsuited to the present day
Banking models are still based on :
- 3-year reviews
- Company seniority
- Standard ratios (equity, debt, WCR)
- A standardized reading of sector risk
A start-up or a fast-growing SME :
- May not have complete history
- Invests heavily in R&D (to the detriment of the bottom line)
- Presents an atypical... but healthy profile
📌 Result: a distorted score, restricted access to financing.
🎯 Rigid scoring logic with little contextualization
Classic models :
- Apply the same criteria to all sectors
- Do not take into account subscription, recurrence, or potential
- Do not integrate recent behavioral data
Example:
A SaaS company with €10k/month MRR revenues, 0 debts and 2 balance sheets, may be "risky" according to a bank.
A traditional merchant with little inventory but 5 balance sheets is considered "solvent".
🧨 The scoring is then out of sync with the reality on the ground.
What does AI scoring based on real-time data offer?
🤖 A dynamic, connected and contextual approach
Modern scoring engines like RocketFin leverage :
- Data updated in real time (KYC, status, INPI listing)
- Weak behavioral signals (unpaid suppliers, legal anomalies, frequency of bank consultations)
- Machine learning models
✅ This enables a 360° assessment, much closer to the company's actual profile.
🧠 The role of machine learning
AI models (Gradient Boosting, Random Forest, etc.):
- Learn from previous cases
- Tailor the weight of each criterion to each sector
- Detect combinations of weak signals not visible to the human eye
Example:
A company with a good balance sheet, but 3 changes of manager, an inactive SIREN on INSEE and an inconsistent address = a high-risk dossier.
The AI will spot this even if the figures seem "good".
Case study: AI Scoring vs. Bank Scoring on a Tech SME
Criteria | Bank | AI RocketFin |
---|---|---|
Balance sheet 2022 | OK | OK |
Seniority (2 years) | Penalized | Neutral |
Low net income | Penalized | Weighted with MRR |
Valid SIREN, actual activity | Don't look | Taken into account |
Experienced manager | Non-valued | Integrated into the score |
Stable supplier payments | Not included | Integrated (via behavioral score) |
Final bank score : 48/100 → Refused
RocketFin score : 78/100 → Accepted automatically
How to move from bank scoring to agile scoring
🔄 Transition to AI scoring
-
Identify your current blocking criteria
(which "good" files are being unfairly rejected?) -
Connect your internal/external data flows
(banks, invoices, legal status, APIs) -
Define adaptable business rules
by type (VSE, SaaS, agricultural sector, etc.) -
Choose a plug & play AI scoring engine
like RocketFin, with API + human interface -
Measure the business impact of change
(gain in acceptance rate, speed, reliability)
5 common mistakes in corporate credit scoring
- Apply the same rules to all files
- Based solely on the past (balance sheet)
- Not integrating behavioral data
- Ignore weak legal signals (e.g.: managers, SIREN)
- Refuse without traceability or clear justification
Concrete benefits of AI scoring in B2B operations
Profit | Impact |
---|---|
Decision in less than 10 sec | Friction-free processing |
+30 to +50% acceptance | Growing business |
Reduction of false negatives | Fewer good customers lost |
Full traceability RGPD/LCB-FT | Enhanced compliance |
No need for a dedicated scoring team | Guaranteed scalability |
FAQ - Corporate credit scoring
Are AI models auditable?
Yes, RocketFin offers justified scoring, with logs, weightings and documented alerts.
Is scoring customizable?
Absolutely. By sector, business volume, type of risk accepted, etc.
Is it RGPD-compliant?
Yes, with explicable rules, adjustable thresholds, human proofreading possible.
🔗 Read more
👉 Find out how RocketFin is redefining B2B credit scoring: www.rocketfin.ai
🟢 Would you like to test RocketFin in advance?
Request your access here