How to detect fraud and inconsistencies in pro financing files
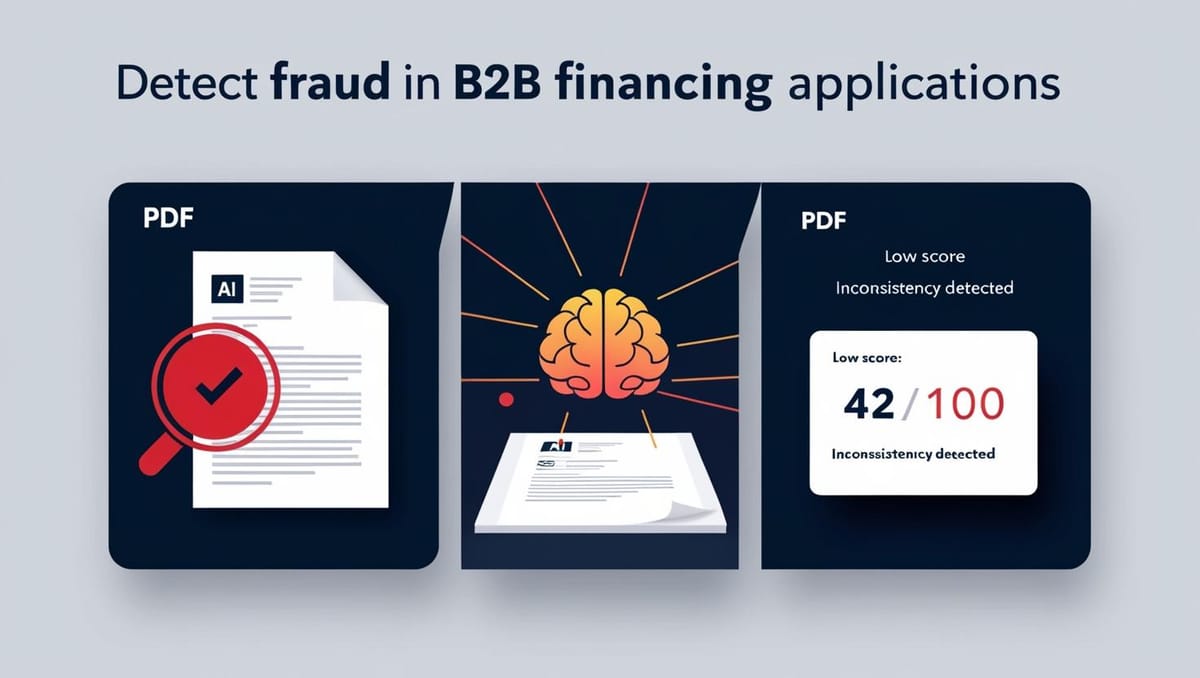
Document fraud is a growing risk in B2B financing. Between falsified documents, legal inconsistencies and fictitious activities, professional credit players must redouble their vigilance.
But faced with growing volumes of cases, how do you spot these weak signals without mobilizing an army of analysts?
The answer lies in a combination of automated business rules and artificial intelligence applied to fraud detection.
Overview of common frauds in professional files
🧾 1. falsification of balance sheets or income statements
- Using fake, hand-generated PDFs
- Manipulating ratios to mask losses
- Reuse of older documents as "recent versions
Example: a 2022 balance sheet modified with a new sales figure, but still bearing the 2021 publication date.
🏢 2. legal or administrative inconsistencies
- Inactive or unregistered SIREN
- Declared manager not complying with legal requirements
- Fictitious address or business mailbox
- Declaration of an activity different from the articles of association
🔗 3. Capital manipulations
- Companies linked together in the same billing network
- Joint manager of several structures in difficulty
- Change of manager or shareholder just prior to application
📄 4. False proof of income or contract
- Falsified payslips for salaried executives
- False partnership contracts to boost order books
- Intra-group loans disguised to artificially improve cash flow
Why human detection is no longer enough
Even the best analysts can miss the point:
- From a cleanly modified PDF
- From an executive already reported in another case
- A deactivated SIREN between two checks
And above all:
- They don't have the time to analyze thousands of cross-referenced signals per file.
- Fraud is evolving fast, sometimes generated by AI or no-code tools (false Kbis, false bank statements).
👉 Automation is becoming essential to cope with the complexity of modern fraud.
How AI and business rules detect fraud effectively
🤖 Step 1: real-time cross-checks
Examples of possible automations :
- Compare consistency between SIREN and recent articles of association
- Check that the manager is registered with Pappers/Infogreffe
- Cross-reference tax data with legal form (e.g. no VAT for auto-entrepreneurs)
🧠 Step 2: Anomaly detection with AI
AI identifies :
- Suspicious behavior based on thousands of past cases
- Recurring reasons for fraud (e.g. change of manager, dormant status, data inconsistencies)
- Combinations of otherwise invisible weak signals
🔍 Example: a "new" company, with an empty building address, high share capital but no employees = suspicious profile.
⚖️ Step 3: reliability scoring + alert
Each file can be graded according to :
- Documentary consistency
- Structural strength
- Its legal legitimacy
- History of the company
With RocketFin :
- Les dossiers à forte incohérence (< 50/100) sont automatiquement refusés
- Suspicious or ambiguous files (50-70) go through human validation
- Reliable and consistent files (> 70) can be automatically accepted.
Case study: automated detection in a financing platform
A B2B platform handling 500 requests/month integrated RocketFin. Result after 3 months:
- 🚫 27 fraud attempts detected automatically (false statuses, inconsistent balance sheets)
- ⏱️ Automatic processing of 65% of files without an analyst
- 🧠 Improved reliability of accepted files: +34%.
- 📉 Decrease in 30-day delinquencies: -21%.
Best practices for strengthening your anti-fraud strategy
- Integrate dynamic business rules (by sector, legal form, declared sales)
- Automate document reading as soon as the file is received
- Check the history of managers and SIRENs via open sources
- Scoring inter-document consistency (same social capital on all media?)
- Set up a human validation system for weak signals
Common mistakes to avoid
- Rely solely on the PDF's appearance (design ≠ reliability)
- Overlook minor inconsistencies (often revealing)
- Ignore capital links between entities
- Don't log checks performed
- Accept borderline files without audit trail
FAQ - Fraud detection and B2B scoring
Can falsified documents be detected automatically?
Yes, by analyzing content consistency, file metadata and inconsistencies with other sources.
Can AI detect fraudulent schemes?
It can't replace an analyst in an in-depth investigation, but it can alert you to weak signals or repeat offenders (same manager, same address...).
Is it legal to score the reliability of a file?
Yes, as long as the assessment is objective and non-discriminatory, and as long as a human review is possible.
🔗 Read more
👉 Find out how RocketFin secures your B2B financing decisions with intelligent inconsistency detection: www.rocketfin.ai
🟢 Request your RocketFin preview access
Access form